Case Study
ML-Powered Image Processing for PDF Digitization and Home Self-Monitoring of Medical Data
About
An emerging organization offers a robust machine learning (ML) platform that leverages image processing and Generative AI to digitize PDF medical reports, including data from approved home monitoring devices such as ECGs, blood pressure monitors, HbA1c tests, blood glucose monitors, SpO2 sensors, and more. The platform simplifies self-monitoring for patients, helping them and their healthcare providers better manage vital health indicators by providing insights and health trends. It also integrates seamlessly with healthcare systems to enhance clinical decision-making.
Challenges
-
Complexity of Medical Data:
-
Medical PDFs such as ECGs, lab reports, and device readouts come in various formats, making it challenging to standardize data extraction. Capturing reliable data from these varied sources was a significant challenge, especially when integrating self-monitored health data from unconnected devices.
-
-
Image Quality and Variability:
-
Medical PDFs and images often contain handwritten notes, scanned documents, and varying resolutions, requiring sophisticated preprocessing and noise reduction techniques.
-
-
Manual Processes:
-
Traditionally, patients and caregivers had to manually log or share their readings during visits, which delayed real-time care and often led to fragmented data.
-
-
Security and Compliance:
-
Ensuring the privacy and security of sensitive health data was essential, particularly when digitizing personal health records for integration into electronic medical systems. HIPAA and GDPR compliance were key priorities.
-
Our solutions
-
Machine Learning for Document Parsing and Data Extraction:
-
We deployed custom ML and LLM models to digitize and parse a wide variety of medical reports, including device-generated data from ECGs, blood pressure monitors, HbA1c tests, and more. Custom optical character recognition (OCR) and AI-based advanced object recognition techniques were implemented to detect and digitize images, text, and annotations commonly found in medical reports. The models handled low-quality scans and varied formats to extract relevant data with over 95% accuracy.
-
-
Image Preprocessing and Enhancement:
-
Image processing techniques were applied to clean and enhance the quality of scanned documents. This included noise reduction, deblurring, and contrast adjustment to ensure clearer data extraction, even from low-quality scans.
-
-
Error-Handling and Validation:
-
A validation layer was added to the data extraction process to flag potential errors or anomalies in the digitized data. Machine learning algorithms were trained to detect outliers or inconsistent values, which could then be manually reviewed or corrected.
-
-
Self-Monitoring at Home Integration:
-
The platform simplified home-based self-monitoring by enabling users to easily submit their vital readings. Patients could take a reading using any reliable device, snap a photo of the device’s screen, or share a PDF of their ECG report via email or WhatsApp. This eliminated the need for an app or Bluetooth connection, making the system accessible for all users.
-
-
Medical Practice Integration:
-
The platform enabled healthcare providers and practices to integrate patients' self-monitoring data directly into their practice portals. This integration streamlined data flow from home monitoring devices into the clinic's electronic health records (EHRs), improving patient outcomes and facilitating higher value-based reimbursement.
-
-
Data Security and HIPAA Compliance:
-
Strong encryption protocols and access controls were implemented to ensure the privacy and security of sensitive medical information. The platform was built with HIPAA and GDPR compliance in mind, protecting patient data from unauthorized access and breaches.
-
Outcome and Metrics of Success:
-
Increased Efficiency and Accuracy:
-
The ML-powered platform significantly reduced the time required to digitize and process medical PDFs, providing near real-time access to medical data. Healthcare providers saw a huge improvement in document processing speed compared to manual data entry methods. Achieved best-in-market accuracy in data extraction from medical PDFs, including device readouts and lab reports, leading to more reliable data for clinical decisions.
-
-
Improved Data Integration:
-
By enabling seamless integration with EMR systems, we were able to help client facilitate smoother workflows for healthcare providers, ensuring that digitized medical data could be quickly and easily accessed for patient care, clinical reviews, and medical research.
-
-
Empowering Self-Monitoring at Home:
-
Simplified self-monitoring at home, providing a hassle-free experience for users. Without the need for an app or Bluetooth, patients could use any FDA-cleared or AMA-validated device to track their health, improving health vigilance across the board. As a result, users felt more empowered to take control of their health, with their comprehensive self-monitored records enabling more informed discussions with their primary care providers.
-
-
Seamless Data Integration and Access:
-
The digitized data was made accessible through the platform’s secure Dashboard, where patients and caregivers could share detailed reports with medical professionals. This real-time access improved clinical decision-making and reduced delays in care.
-
-
Accessibility and Affordability:
-
The service, which offers basic functionality free of charge, made home self-monitoring accessible to a wider audience to provide affordable, easy-to-use health monitoring solutions allowing users from various backgrounds to track their health metrics easily, making it an inclusive solution for home, work, and community settings.
-
-
Improved Clinical Outcomes:
-
By integrating self-monitoring data with clinic and hospital systems, the platform supported initiatives like Decentralized Clinical Trials, Hospital-at-Home programs, and community health efforts to provide offer timely, remote care while improving patient outcomes and enhancing value-based reimbursement.
-
-
Scalability and Global Reach:
-
The ML-driven solution demonstrated scalability, handling data from various home monitoring devices across diverse settings. Its success in self-monitoring programs worldwide represented a step toward the future of affordable healthcare, supporting millions of individuals in maintaining their health at home.
-
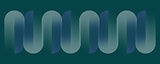
Get started with Novarroh Today